Understanding the True Cost of Large Language Models : Short Executive Perspective series by Rohan Sharma
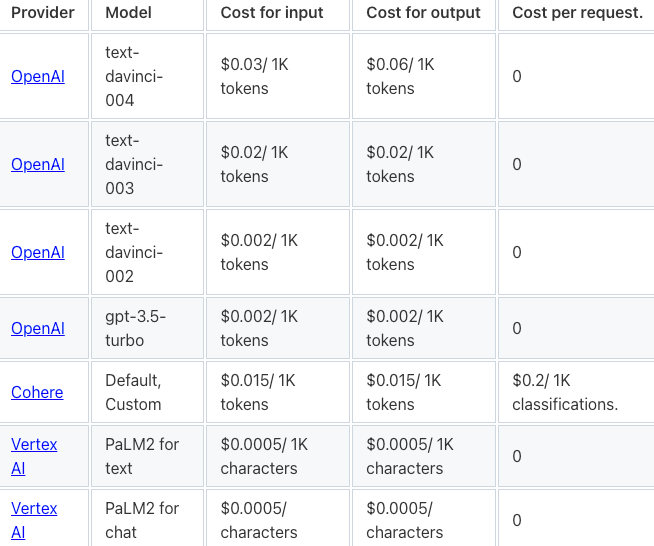
I have had dozens of conversations with CIO’s, CDO’s over the last 12 month at numerous AI summits, conferences and one of the top agenda item is cost to deploy AI into their organizations. So writing my short perspective on what options do C-suite, CIO’s have in deploying LLM’s and what does it roughly cost for each option.
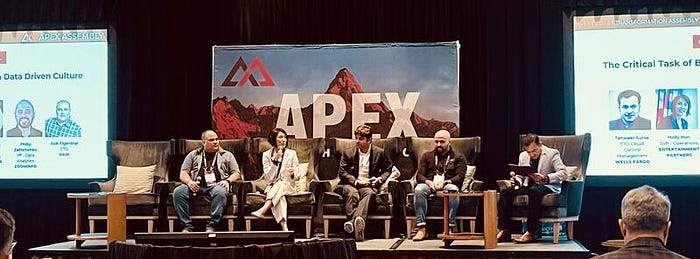
Key for AI practitioners is the cost of integrating LLM applications, with two main options:
- API Access Solution: Using closed-source models like GPT-4 via API.
- On-Premises Solution: Building and hosting a model from an open-source base in-house.
This article explores the cost components of LLM applications:
- Cost of Project Setup and Inference.
- Cost of Maintenance.
- Other Associated Costs.
Initial Setup and Inference Costs:
- API access involves charges for input and output tokens, and sometimes per request, with prices varying by provider and use case, so it’s a “per request pricing model”
- On-premises setup requires a robust IT infrastructure, with costs depending on the model size and the chosen hardware, billed hourly, so it’s a “ hourly billing pricing model”.
Maintenance Costs:
- API access solutions may include fine-tuning services in their pricing.
- On-premises solutions incur costs for IT infrastructure used for retraining.
Cost Comparison:
The article presents a hypothetical chatbot API project. Daily token usage for a chatbot with 50 discussions of 1000 words each is 37,500 tokens, costing $0.75 per day or $270 annually with GPT-3.5. Costs increase linearly with request volume.
API Access Option
Starting with API access option, OpenAI, Cohere& Vertex AI, are 3 most commonly used providers for access to their models through an API, which operates on a token-based system.
Every provider offers varied pricing models based on the particular application (like summarization, classification, embedding, etc.) and the selected model. Charges are incurred for each request, calculated on the basis of the total input tokens (input cost) and output tokens generated (output cost). Additionally, certain scenarios might involve a charge per request. As an instance, using Cohere’s models for classification tasks incurs a fee of $0.2 per 1,000 classifications.
Here’s the breakdown: ‘prompt tokens’ are your conversation starters, and ‘sampled tokens’ are the AI’s responses.
💰The cost? $0.03 per 1,000 prompt tokens and double that for the sampled tokens.So with that calculation for a hypothetical chatbot API project, daily token usage for a chatbot with 50 discussions of 1000 words each is 37,500 tokens, costing $1.11 per day or $33/month or $400/annum.
GPT-3.5 costs increase linearly with request volume.
Small LLMs: The Budget-Friendly Choice
There’s an alternative route — smaller LLMs. These models, boasting 7–15 billion parameters, are like the compact cars of the AI world. Great for general tasks but might not hit the mark for more specialized needs.
Small-sized models can be run run locally on your personal computers
The Grandeur of Gigantic LLMs
On the flip side, we have the Goliaths of the LLM world like LLAMA 2 65B parameters. These LLM’s can dissect and understand complex queries with remarkable accuracy but at a premium cost.Large-sized models require hosting on cloud servers such as AWS, Google Cloud Platform, or your internal GPU server.
The Cost of computational Power
Pricing in this context is determined by the hardware, and users are billed on an hourly basis.
The cost varies, with the most affordable option being $0.6 per hour for a NVIDIA T4 (14GB) and the highest costing $45.0 per hour for 8 NVIDIA A100 GPUs (640GB).
Running a smaller LLM might require a GPU, like the NVIDIA T4 (14GB), costing approximately $0.6 per hour or $450/month or $5500/annum.
If you opt for a larger model, we might need to use 8 NVIDIA A100 GPUs (640GB), and expenses could soar to $25–45/hour roughly translating to around $18,000- $32,000/month or $250K-$4500k.
These costs only account for GPU usage, without considering other associated expenses like storage , data transfer, and potential fine tuning processes
In Summary
The world of LLMs is a landscape of choices, each with its own cost-benefit analysis.
Understanding the cost implications is key for any Enterprise / startup looking to harness the power of AI.
#AIcost #LLMcost #TotalCostofOwnership #TCO #LLMcostsavings
#Costs #ITBudgets
Hi, this is a comment.
To get started with moderating, editing, and deleting comments, please visit the Comments screen in the dashboard.
Commenter avatars come from Gravatar.